Cardiovascular disease (CMDs) accounted for 17.5 million deaths in 2012. Coronary artery disease (CAD) and stroke are the primary diseases. Franzén et al. use Genome-wide Association Studies (GWAS) to identified downstream gene-regulatory mechanisms associated with risk for CMDs. They share their findings in Science Magazine (Science 19 Aug 2016: Vol. 353, Issue 6301, pp. 827-830 DOI: 10.1126/science.aad6970).
Creative Biomart can provide you kinds of molecular tools such as recombinant Cardiovascular Proteins for you research application about CMDs and CADs. Here is this article for you:
GWAS loci typically span large, noncoding, intergenic regions with numerous single-nucleotide polymorphisms (SNPs) in strong linkage disequilibrium. These regions are enriched in cis–regulatory elements and expression quantitative trait loci (eQTLs), suggesting that gene regulation is the principal mechanism by which risk loci affect complex disease etiology. However, it is largely unknown whether this gene-regulatory effect includes one or several genes acting in one or multiple tissues and whether risk loci for different diseases share cis- and trans–gene regulation. A better understanding of gene regulation may also shed light on why known GWAS risk loci explain only ~10% of expected heritable variance in CMD risk. Possibly, multiple risk loci, acting through common cis- and trans-genes, contribute synergistically to heritability.
In the Stockholm-Tartu Atherosclerosis Reverse Networks Engineering Task study (STARNET), we recruited 600 well-characterized CAD patients; genotyped DNA (6,245,505 DNA variant calls with minor allele frequency >5%); and sequenced RNA isolated from blood, atherosclerotic-lesion-free internal mammary artery (MAM), atherosclerotic aortic root (AOR), subcutaneous fat (SF), visceral abdominal fat (VAF), skeletal muscle (SKLM), and liver (LIV) (15 to 30 million reads per sample).
In total, ~8 million cis-eQTLs were identified, and nearly half were unique SNP-gene pairs. The STARNET cis–eQTLs were enriched in genetic associations established by GWAS for CAD, CMDs, and Alzheimer’s disease (AD) and were further enriched after epigenetic filtering. Of 3326 genome-wide significant-risk SNPs identified by GWAS to date, 2,047 (61%) had a matching cis–QTL in STARNET (Fig. 1A). Of the 54 lead risk SNPs verified in meta-analyses of CAD GWAS, 38 cis-eQTLs with a regulatory trait concordance score (RTC) >0.9 and at least one candidate gene were identified in STARNET. Compared with large data sets of cis-eQTL isolated only from blood, cis-eQTLs across all tissues in STARNET matched >10-fold more CAD and CMD-related GWAS risk SNPs (Fig. 1B). STARNET cis-eQTLs isolated from CAD-affected tissues also matched several-fold more CAD and CMD-related GWAS risk SNPs than cis-eQTLs from corresponding tissues isolated from predominantly healthy individuals in the Genotype Tissue Expression (GTEx) study (Fig. 1C). Thus, not all gene-regulatory effects of disease-risk SNPs are identifiable in blood or healthy tissues. This notion was further underscored by comparing the statistical significances of cis-eQTLs for GWAS risk SNPs in STARNET with corresponding associations in GTEx (Fig. 1D). In STARNET, gene fusions and CAD-related loss of function mutations were also detected.

The cis effects of disease-associated risk loci identified by GWAS are central for understanding downstream molecular mechanisms of disease. However, these cis-genes likely also affect downstream trans–genes. To identify possible trans effects, we ran a targeted analysis to call both cis– and trans-genes for lead risk SNPs identified by GWAS. After assigning cis–eQTLs for 562 risk SNPs for CAD, CMDs, and AD, we used a causal inference test to conservatively call causal correlations between the cis–genes and trans-genes by assessing the probability that an interaction was causal [SNP→cis-gene→trans–gene; false discovery rate (FDR) < 1%] and not reactive (SNP→trans-gene→cis-gene; P > 0.05). We found extensive sharing of cis– and trans-gene regulation by GWAS risk loci across tissues and CMDs. In CAD, 28 risk loci with at least one causal interaction (FDR < 1%, P > 0.05) had a total of 51 cis–genes and 1040 trans-genes. Of these, 26 risk loci, 37 cis–genes [including 27 key drivers], and 994 trans-genes were connected in a main CAD regulatory gene network acting across all seven tissues (Fig. 2). The trans-genes in this network were enriched with genes previously associated with CAD and atherosclerosis (Fisher’s test, 1.54-fold; P = 8 × 10–10 ) (table S11). Sharing of cis/trans-genes downstream of complex disease risk loci also emerged for other CMDs and AD. In fact, we identified 33 cis-genes regulated by risk SNPs across all CMDs, including CAD and AD, acting as key drivers in a pan-disease cis/trans-gene regulatory network (Fig. 3A).

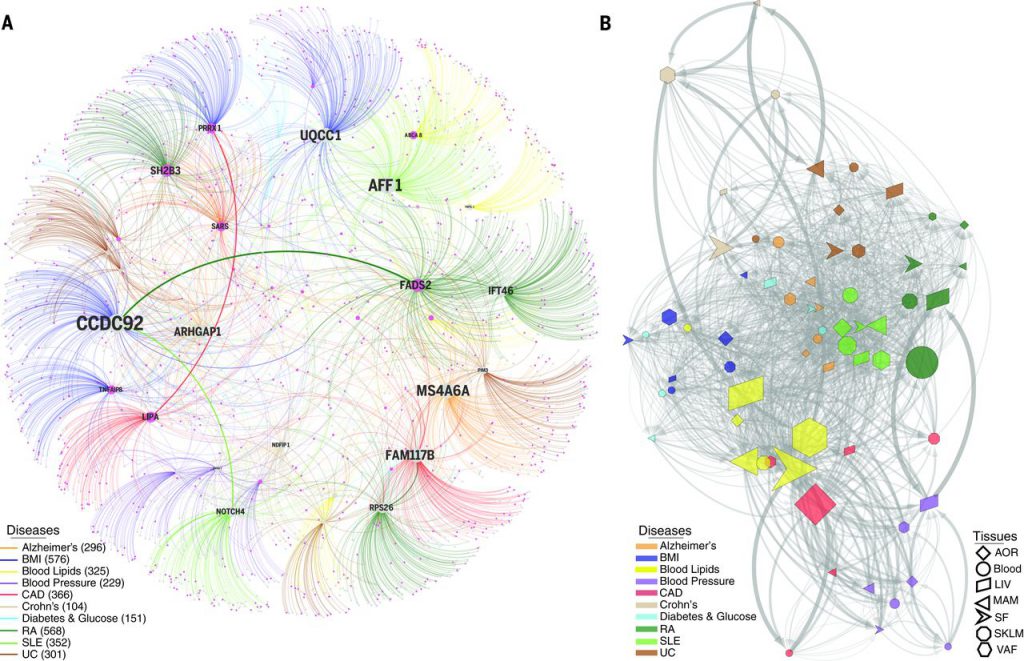
Among CMDs, cis/trans-genes of GWAS risk SNPs for blood lipid levels emerged as central (Fig. 3B) where tissue-specific downstream effects were, besides LIV (46 cis- and 150 trans-genes), observed in the fat tissues (SF, 45 cis– and 372 trans-genes; VAF, 38 cis- and 465 trans-genes). Visceral abdominal fat examples included ABCA8/ABCA5(rs4148008) associated with 36 downstream trans-genes in VAF and HDL (high-density lipoprotein); EVI5 (rs7515577) associated with 32 VAF trans-genes and total cholesterol; andSTARD3 (rs11869286) associated with 7 VAF trans–genes and HDL. In addition, the cis-geneTMEM258 (rs174546) with 22 trans-genes in abdominal fat surfaced as a parallel/alternative regulatory site of plasma low-density lipoprotein (LDL) to the proposed FADS-1,2,3 in LIV. Other risk SNPs with VAF-specific cis-genes had few or even no trans–genes. For example, two risk SNPs—rs11206510 for CAD and rs12046679 for LDL cholesterol level—regulate PCSK9 in VAF, not in LIV (Fig. 4, A and B). The VAF specificity of these eQTLs PCSK9was confirmed in an independent gene expression data set from morbidly obese patients (Fig. 4C), suggesting that PCSK9 is secreted from VAF into the portal vein to affect hepatic LDL receptor degradation, LDL plasma levels, and risk for CAD. Interestingly, and as previously suggested, we observed that STARNET patients in the upper, compared to the lower, 5th to 20th percentiles of waist-hip ratio (i.e., patients with and without “male fat”) had higher levels of circulating PCSK9 (Fig. 4D) and LDL/HDL ratio (Fig. 4E).

STARNET provides new insights into tissue-specific gene-regulatory effects of disease-associated risk SNPs identified by GWAS, as exemplified by abdominal fat for blood lipids, and will be a complementary resource for exploring GWAS findings moving forward. Furthermore, STARNET also revealed unexpected sharing of cis- and trans-genes downstream of risk loci for CMDs across both tissues and diseases. We anticipate that the identified cis/trans-gene regulatory networks will help elucidate the complex downstream effects of risk loci for common complex diseases, including possible epistatic effects that could shed light on the missing heritability of CMD risk. Given the detailed phenotypic data on STARNET patients, we can begin to identify how genetic variability interacts with environmental perturbations across tissues to cause pathophysiological alterations and complex diseases.
[table caption=”Related Products” width=”800″ colwidth=”80|380|180″ colalign=”left|left|center|center|center”]
NO.,Production Classification
1,Cardiovascular Proteins
2,Vasculature Proteins
3,Angiogenesis Proteins
4,Cardiovascular Disease Therapeutic Targets Proteins
[/table]